DATA ANNOTATION
What is Data Annotation?
For AI to do the amazing things it does, like recognising faces in photos, understanding speech, or predicting the weather, it needs to be trained on annotated (labelled or tagged) data.
Data annotation means adding labels or tags to the data so that AI can learn from it. When you have a picture of a dog, the AI model doesn’t know it’s a dog unless someone tells it. By adding a label like "dog" to the picture, the AI model can start recognising dogs in other images. Data annotation is like labelling every item in a classroom so the AI model can understand what that is. Without labelled data, AI wouldn’t know how to tell the difference between a cat and a dog in a picture or what someone is saying in a voice recording.
Types of Data Annotation
Some common examples:
Image Annotation: To enable an AI model to recognise objects or people in photos, the objects in each image need labels/tags. To illustrate, if you have a picture of a dog, you would label it "dog," and if you have a picture of a cat, you would label it "cat." Drawing boxes around objects like cars or traffic signs is also a form of annotation to help the AI model learn how to identify them.
Text Annotation: In text annotation, words or sentences are labelled to help the AI model understand language. This might include labelling parts of speech (like nouns or verbs), identifying the sentiment (whether a sentence is positive or negative), or tagging names of places, people, or things.
Audio Annotation: In audio annotation, sounds, speech, or music are labelled so that the AI model can learn to recognise them. This could include labelling a sound as "dog barking" or "music playing" or even tagging specific words in a voice recording.
Video Annotation: Video annotation is used for training AI models to understand movement and actions in videos. This might include labelling activities like walking, running, driving, or identifying different objects that appear in each frame.
Contact
contact@thedatatrainers.com
+61 (2) 8375 2707
© 2025. All rights reserved.
Follow Us:
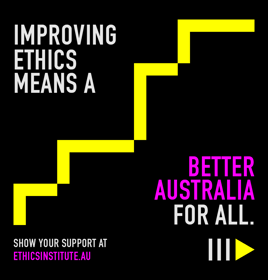
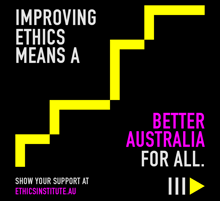